Roboflow Universe
Analyze any computer vision datasets from Roboflow Universe.
Install fastdup
First, install fastdup and verify the installation.
pip install fastdup
Now, test the installation by printing the version. If there's no error message, we are ready to go.
import fastdup
fastdup.__version__
'1.38'
Roboflow Universe
Roboflow Universe hosts over 200,000 computer vision datasets.
In order to download datasets from Roboflow Universe, sign-up for free.
Now, head over to https://universe.roboflow.com/ to search for the dataset of interest.
Once you find a dataset, click on the 'Download Dataset' button on the dataset page.
A pop-up will appear with a code snippet to download the dataset into your local machine. Copy the code snippet.
Warning
The code snippet consists of an API key that is tied to your account. Keep it private.
Install Roboflow Python
The Roboflow Python Package is a Python wrapper around the core Roboflow web application and REST API. To install, run:
pip install roboflow
Now you can use the Roboflow Python package to download the dataset programmatically into your local machine.
from roboflow import Roboflow
rf = Roboflow(api_key="YOUR_API_KEY")
API Key
Replace
YOUR_API_KEY
with your own API key from Roboflow. Do not share this key beyond your team, it contains a private key that is tied to your Roboflow account.
Download Dataset
For this tutorial, let's download the Dash Diet 101 Dataset in COCO annotations format into our local folder.
project = rf.workspace("dash101").project("dash-diet-101")
dataset = project.version(4).download("coco")
Once completed, you should have a folder in your current directory with the name DASH-DIET-101-4
.
The DASH-DIET-101 dataset was created by Bhavya Bansal, Nikunj Bansal, Dhruv Sehgal, Yogita Gehani, and Ayush Rai with the goal of creating a model to detect food items that reduce Hypertension.
It contains 16,900 images of 101 popular food items with annotated bounding boxes.
Analyze Bounding Boxes with fastdup
To run fastdup, you only need to point input_dir
to the folder containing images from the dataset.
fd = fastdup.create(input_dir='./DASH-DIET-101-4/train')
fastdup works on both labeled and unlabeled datasets. Since this dataset is labeled, let's make use of the labels by passing them into the run
method.
fd.run(annotations='DASH-DIET-101-4/train/_annotations.coco.json')
Now sit back and relax as fastdup analyzes the dataset.
Invalid Bounding Boxes
Since this dataset is annotated with bounding boxes, let's check if all the bounding boxes are valid.
Bounding boxes that are either too small or go beyond image boundaries are flagged as bad bounding boxes in fastdup.
Let's get the invalid bounding boxes.
fd.invalid_instances()
col_x | row_y | width | height | label | crop_filename | filename | index | is_valid | |
---|---|---|---|---|---|---|---|---|---|
20121 | 0 | 27 | 413.638 | 388.924 | Kimchi | work_dir/crops/DASH-DIET-101-4train68747470733a2f2f73332e616d617a6f6e6177732e636f6d2f776174747061642d6d656469612d736572766963652f53746f7279496d6167652f6j474b624b4c50427234665344673d3d2d3435393632393038312e313464656435353538386337_jpg.rf.9676c410f32974f8bd53b6c47db156e5.jpg_0_27_413_388.jpg | DASH-DIET-101-4/train/68747470733a2f2f73332e616d617a6f6e6177732e636f6d2f776174747061642d6d656469612d736572766963652f53746f7279496d6167652f6j474b624b4c50427234665344673d3d2d3435393632393038312e313464656435353538386337_jpg.rf.9676c410f32974f8bd53b6c47db156e5.jpg | NaN | False |
20181 | 20 | 0 | 390.749 | 415.651 | Kimchi | work_dir/crops/DASH-DIET-101-4train68747470733a2f2f73332e616d617a6f6e6177732e636f6d2f776174747061642d6d656469612d736572766963652f53746f7279496d6167652f6j474b624b4c50427234665344673d3d2d3435393632393038312e313464656435353538386337_jpg.rf.a4d236ef9262303f7fc5e96b4304715e.jpg_20_0_390_415.jpg | DASH-DIET-101-4/train/68747470733a2f2f73332e616d617a6f6e6177732e636f6d2f776174747061642d6d656469612d736572766963652f53746f7279496d6167652f6j474b624b4c50427234665344673d3d2d3435393632393038312e313464656435353538386337_jpg.rf.a4d236ef9262303f7fc5e96b4304715e.jpg | NaN | False |
20492 | 0 | 20 | 413.601 | 396.000 | Kimchi | work_dir/crops/DASH-DIET-101-4train68747470733a2f2f73332e616d617a6f6e6177732e636f6d2f776174747061642d6d656469612d736572766963652f53746f7279496d6167652f6j474b624b4c50427234665344673d3d2d3435393632393038312e313464656435353538386337_jpg.rf.60d7efa443842a8ea2171337e8b6a49a.jpg_0_20_413_396.jpg | DASH-DIET-101-4/train/68747470733a2f2f73332e616d617a6f6e6177732e636f6d2f776174747061642d6d656469612d736572766963652f53746f7279496d6167652f6j474b624b4c50427234665344673d3d2d3435393632393038312e313464656435353538386337_jpg.rf.60d7efa443842a8ea2171337e8b6a49a.jpg | NaN | False |
import pandas as pd
bad_bb = pd.read_csv('work_dir/full_image_run/atrain_features.bad.csv')
bad_bb
index | filename | error_code | |
---|---|---|---|
0 | 23513 | DASH-DIET-101-4/train/untitled-2-1-_jpg.rf.2cc0804ad0a8d457291b39d72b32ea1f.jpg | ERROR_BAD_BOUNDING_BOX |
1 | 24030 | DASH-DIET-101-4/train/IMG_1282_jpg.rf.e5d454a356160bd444b71e19c9350f72.jpg | ERROR_BAD_BOUNDING_BOX |
2 | 24068 | DASH-DIET-101-4/train/IMG_1282_jpg.rf.f669ecf808bd1721e724ef14c7ede206.jpg | ERROR_BAD_BOUNDING_BOX |
3 | 29989 | DASH-DIET-101-4/train/Almond_13-Original-Size-_jpg.rf.61e38e0da9a2aa99b20ff801c44a47dd.jpg | ERROR_BAD_BOUNDING_BOX |
4 | 35693 | DASH-DIET-101-4/train/carrot_93_jpg.rf.a242faf4608f26c230f476b5feb9f27c.jpg | ERROR_BAD_BOUNDING_BOX |
5 | 47754 | DASH-DIET-101-4/train/pistachios-1570x1047_jpg.rf.9b4218a77ea0530cac6db0abb3491392.jpg | ERROR_BAD_BOUNDING_BOX |
6 | 53836 | DASH-DIET-101-4/train/cabbage-green-50lb-case_jpg.rf.9b8d2677a0a8c7c0d4ceb961cc91aaab.jpg | ERROR_BAD_BOUNDING_BOX |
7 | 53941 | DASH-DIET-101-4/train/cabbage-green-50lb-case_jpg.rf.5459cd9f2b964dc5e84fb29905f95353.jpg | ERROR_BAD_BOUNDING_BOX |
8 | 53945 | DASH-DIET-101-4/train/cabbage-green-50lb-case_jpg.rf.546e265a2b83cd03e7d5d7c98c2d51ef.jpg | ERROR_BAD_BOUNDING_BOX |
9 | 54123 | DASH-DIET-101-4/train/cabbage-green-50lb-case_jpg.rf.6887156a700058674a259ce271ea74fe.jpg | ERROR_BAD_BOUNDING_BOX |
10 | 54323 | DASH-DIET-101-4/train/cabbage-green-50lb-case_jpg.rf.f83f582b75b3ba9ed396004997edc7a2.jpg | ERROR_BAD_BOUNDING_BOX |
11 | 58921 | DASH-DIET-101-4/train/Pomegranates-shutterstock_152360780_jpg.rf.8c5c9586aaa67739a99211a76fc21359.jpg | ERROR_BAD_BOUNDING_BOX |
12 | 59463 | DASH-DIET-101-4/train/Pomegranates-shutterstock_152360780_jpg.rf.71c53b5feee5f6677bddb176380f2b6c.jpg | ERROR_BAD_BOUNDING_BOX |
13 | 70884 | DASH-DIET-101-4/train/OIP-24-_jpg.rf.23fc31cbd35c1de823b99968a19e6d2c.jpg | ERROR_BAD_BOUNDING_BOX |
14 | 71908 | DASH-DIET-101-4/train/90d098b212fc9b6d28ae069e150f8f75_jpg.rf.fadbb69263e413a39ed153caf9d23e0c.jpg | ERROR_BAD_BOUNDING_BOX |
15 | 79343 | DASH-DIET-101-4/train/Potato_38_jpg.rf.2ba6902291f27de4faa2b53291f7a873.jpg | ERROR_BAD_BOUNDING_BOX |
16 | 79370 | DASH-DIET-101-4/train/Potato_38_jpg.rf.3315d9c2535e1b2eb1e1b9045e4979f1.jpg | ERROR_BAD_BOUNDING_BOX |
17 | 85351 | DASH-DIET-101-4/train/Single-Blackberries_28_jpg.rf.465b276617c3780db8a717ce58c49ba9.jpg | ERROR_BAD_BOUNDING_BOX |
18 | 85775 | DASH-DIET-101-4/train/Egg-images_43_jpg.rf.28d73a7161b833d02ef40017086a9948.jpg | ERROR_BAD_BOUNDING_BOX |
19 | 85932 | DASH-DIET-101-4/train/Egg-images_43_jpg.rf.0c240a42a1d5a122bf0df56c6737ce1f.jpg | ERROR_BAD_BOUNDING_BOX |
20 | 85963 | DASH-DIET-101-4/train/Egg-images_43_jpg.rf.0d7f52ff01b12da4a0e1860cef3755fa.jpg | ERROR_BAD_BOUNDING_BOX |
21 | 86632 | DASH-DIET-101-4/train/Egg-images_43_jpg.rf.76de2b114b9b114cfd8262ba6a9b6697.jpg | ERROR_BAD_BOUNDING_BOX |
Let's count the number of images with bad bounding boxes.
bad_bb['error_code'].value_counts()
error_code
ERROR_BAD_BOUNDING_BOX 22
Name: count, dtype: int64
The output shows a total of 22 images contains bounding box issues.
Now it is up to you how to deal with these bounding boxes. You can choose to relabel it or simply discard the entiere image from the training set.
Label Distribution
Let's the label distribution in a bar chart.
Info
This code snippet uses plotly to plot, install plotly with:
pip install plotly
import plotly.express as px
fig = px.histogram(fd.annotations(), x="label")
fig.show()
Bounding Box Size and Shape Issues
Objects come in various shapes and sizes, and sometimes objects might be incorrectly labeled or too small to be useful.
We will now find the smallest, narrowest, and widest objects, and assess their usefulness.
Let's get the annotations and calculate the area and aspect ratio.
df = fd.annotations()
df['area'] = df['width'] * df['height']
df['aspect'] = df['width'] / df['height']
Next, we filter for the smallest 5% bounding boxes and 5% of extreme aspect ratios.
# Smallest 5% of objects:
smallest_objects = df[df['area'] < df['area'].quantile(0.05)].sort_values(by=['area'])
# 5% of extreme aspect ratios
aspect_ratio_objects = df[(df['aspect'] < df['aspect'].quantile(0.05))
|(df['aspect'] > df['aspect'].quantile(0.95))].sort_values(by=['aspect'])
Now let's create a simple function to visualize the images.
from PIL import Image
import matplotlib.pyplot as plt
def plot_image_gallery(df, num_images=5):
# Create 1x5 subplots
fig, axes = plt.subplots(1, num_images, figsize=(15, 3))
# Plot each image in a subplot
for ax, (_, row) in zip(axes, df.iterrows()):
image_path = row['crop_filename']
label = row['label']
# Open the image using PIL
img = Image.open(image_path)
# Display image
ax.imshow(img)
ax.axis('off')
# Set title
ax.set_title(label)
# Show plot
plt.show()
View and visualize the smallest objects DataFrame and plot.
smallest_objects.head()
area | aspect | col_x | row_y | width | height | label | crop_filename | filename | index | is_valid | |
---|---|---|---|---|---|---|---|---|---|---|---|
155 | 150.442810 | 1.346547 | 233 | 121 | 14.233 | 10.570 | Turmeric Powder | work_dir/crops/DASH-DIET-101-4trainR-7-_jpg.rf.0d90e4f32bf1dbd2746f6e58e2c0bb90.jpg_233_121_14_10.jpg | DASH-DIET-101-4/train/R-7-_jpg.rf.0d90e4f32bf1dbd2746f6e58e2c0bb90.jpg | 155.0 | True |
393 | 153.569449 | 0.716413 | 266 | 254 | 10.489 | 14.641 | Turmeric Powder | work_dir/crops/DASH-DIET-101-4trainR-7-_jpg.rf.5389adec93120a33af7fabc41fe89683.jpg_266_254_10_14.jpg | DASH-DIET-101-4/train/R-7-_jpg.rf.5389adec93120a33af7fabc41fe89683.jpg | 393.0 | True |
203 | 155.627325 | 0.774533 | 143 | 168 | 10.979 | 14.175 | Turmeric Powder | work_dir/crops/DASH-DIET-101-4trainR-7-_jpg.rf.846abfdc68392e5eac134dc55631a439.jpg_143_168_10_14.jpg | DASH-DIET-101-4/train/R-7-_jpg.rf.846abfdc68392e5eac134dc55631a439.jpg | 203.0 | True |
490 | 156.648294 | 0.792645 | 116 | 165 | 11.143 | 14.058 | Turmeric Powder | work_dir/crops/DASH-DIET-101-4trainR-7-_jpg.rf.b72fbcbb2776b47eb72ef768fb67d2f8.jpg_116_165_11_14.jpg | DASH-DIET-101-4/train/R-7-_jpg.rf.b72fbcbb2776b47eb72ef768fb67d2f8.jpg | 490.0 | True |
545 | 162.092875 | 1.365055 | 141 | 286 | 14.875 | 10.897 | Turmeric Powder | work_dir/crops/DASH-DIET-101-4trainR-7-_jpg.rf.6162de6a9404060825dfa93613b17879.jpg_141_286_14_10.jpg | DASH-DIET-101-4/train/R-7-_jpg.rf.6162de6a9404060825dfa93613b17879.jpg | 545.0 | True |
plot_image_gallery(smallest_objects)
View the top and bottom-most extreme aspect ratio.
aspect_ratio_objects.head()
area | aspect | col_x | row_y | width | height | label | crop_filename | filename | index | is_valid | |
---|---|---|---|---|---|---|---|---|---|---|---|
1541 | 13374.457902 | 0.087868 | 141 | 26 | 34.281 | 390.142 | Okra | work_dir/crops/DASH-DIET-101-4trainR-14-_jpg.rf.674cbf78ed81a5afc6400d0604a911ae.jpg_141_26_34_390.jpg | DASH-DIET-101-4/train/R-14-_jpg.rf.674cbf78ed81a5afc6400d0604a911ae.jpg | 1541.0 | True |
35552 | 14800.000000 | 0.092500 | 0 | 0 | 37.000 | 400.000 | Carrot | work_dir/crops/DASH-DIET-101-4trainnutrilite-protein-recipes-apple-beet-carrot-juice-cover_jpg.rf.882cec540e8fe015b20e9ac9d4ce8515.jpg_0_0_37_400.jpg | DASH-DIET-101-4/train/nutrilite-protein-recipes-apple-beet-carrot-juice-cover_jpg.rf.882cec540e8fe015b20e9ac9d4ce8515.jpg | 35548.0 | True |
1306 | 14365.950291 | 0.093047 | 144 | 22 | 36.561 | 392.931 | Okra | work_dir/crops/DASH-DIET-101-4trainR-14-_jpg.rf.4bd10c5235e42202a3a0c98af14f0562.jpg_144_22_36_392.jpg | DASH-DIET-101-4/train/R-14-_jpg.rf.4bd10c5235e42202a3a0c98af14f0562.jpg | 1306.0 | True |
1540 | 14533.513197 | 0.095632 | 172 | 26 | 37.281 | 389.837 | Okra | work_dir/crops/DASH-DIET-101-4trainR-14-_jpg.rf.674cbf78ed81a5afc6400d0604a911ae.jpg_172_26_37_389.jpg | DASH-DIET-101-4/train/R-14-_jpg.rf.674cbf78ed81a5afc6400d0604a911ae.jpg | 1540.0 | True |
1305 | 15548.185098 | 0.100660 | 175 | 21 | 39.561 | 393.018 | Okra | work_dir/crops/DASH-DIET-101-4trainR-14-_jpg.rf.4bd10c5235e42202a3a0c98af14f0562.jpg_175_21_39_393.jpg | DASH-DIET-101-4/train/R-14-_jpg.rf.4bd10c5235e42202a3a0c98af14f0562.jpg | 1305.0 | True |
plot_image_gallery(aspect_ratio_objects.head())
plot_image_gallery(aspect_ratio_objects.tail())
Visualize Issues with fastdup Gallery
There are several other methods we can use to inspect and visualize the issues found.
fd.vis.duplicates_gallery() # create a visual gallery of duplicates
fd.vis.outliers_gallery() # create a visual gallery of anomalies
fd.vis.component_gallery() # create a visualization of connected components
fd.vis.stats_gallery() # create a visualization of images statistics (e.g. blur)
fd.vis.similarity_gallery() # create a gallery of similar images
Duplicates & Near-duplicates
First, let's visualize the duplicate images at the bounding box level.
Note
The duplicates visualized here is at the bounding box level, NOT at the image level.
In other words, the bounding box image is cropped from the original image and compared to other bounding box images for duplicates.
fd.vis.duplicates_gallery()
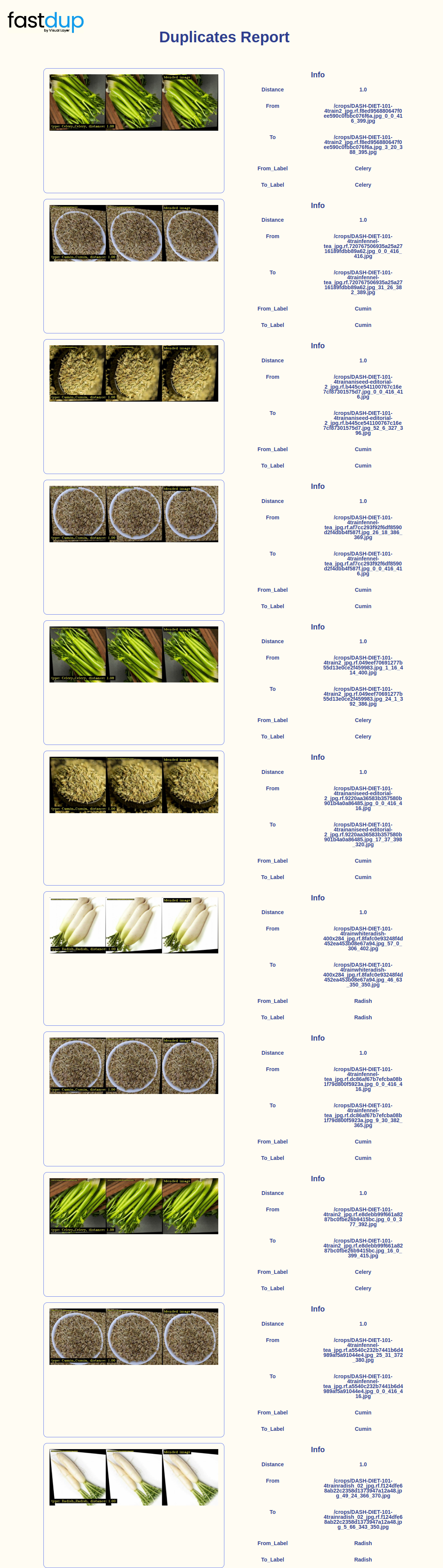
Now let's get the number of exact/near duplicates.
similarity_df = fd.similarity()
near_duplicates = similarity_df[similarity_df["distance"] >= 0.98]
near_duplicates = near_duplicates[['distance', 'crop_filename_from', 'crop_filename_to']]
near_duplicates.head()
distance | crop_filename_from | crop_filename_to | |
---|---|---|---|
0 | 1.0 | work_dir/crops/DASH-DIET-101-4train2_jpg.rf.f8ed956880647f0ee590c0fbbc076f6a.jpg_0_0_416_399.jpg | work_dir/crops/DASH-DIET-101-4train2_jpg.rf.f8ed956880647f0ee590c0fbbc076f6a.jpg_3_20_388_395.jpg |
1 | 1.0 | work_dir/crops/DASH-DIET-101-4train2_jpg.rf.e8debb99f661a8287bc0fbe26b9415bc.jpg_0_0_377_392.jpg | work_dir/crops/DASH-DIET-101-4train2_jpg.rf.e8debb99f661a8287bc0fbe26b9415bc.jpg_16_0_399_415.jpg |
2 | 1.0 | work_dir/crops/DASH-DIET-101-4train2_jpg.rf.e8debb99f661a8287bc0fbe26b9415bc.jpg_16_0_399_415.jpg | work_dir/crops/DASH-DIET-101-4train2_jpg.rf.e8debb99f661a8287bc0fbe26b9415bc.jpg_0_0_377_392.jpg |
3 | 1.0 | work_dir/crops/DASH-DIET-101-4train2_jpg.rf.c2506f976a065ee2f23c30a07a4c349e.jpg_12_38_401_377.jpg | work_dir/crops/DASH-DIET-101-4train2_jpg.rf.c2506f976a065ee2f23c30a07a4c349e.jpg_1_0_415_399.jpg |
4 | 1.0 | work_dir/crops/DASH-DIET-101-4train2_jpg.rf.c2506f976a065ee2f23c30a07a4c349e.jpg_1_0_415_399.jpg | work_dir/crops/DASH-DIET-101-4train2_jpg.rf.c2506f976a065ee2f23c30a07a4c349e.jpg_12_38_401_377.jpg |
Let's see how many near duplicates we find by checking the number of rows in the DataFrame.
len(near_duplicates)
20394
A distance
value of 1.0
indicates an exact duplicate.
As a sanity check, let's show the images that are flagged as duplicates here.
from IPython.display import Image
Image(filename="work_dir/crops/DASH-DIET-101-4trainR-1_jpg.rf.f5a1151cb95e5bbc7d04efb70c805ab0.jpg_193_107_208_215.jpg")
Image(filename="work_dir/crops/DASH-DIET-101-4trainR-1_jpg.rf.00f7c3e0c3b443aa1b4b5a04dc6f26eb.jpg_180_101_216_209.jpg")
Image Clusters
We can also view similar-looking images forming clusters.
fd.vis.component_gallery()

Bright/Dark/Blurry Images
We can show the brightest images from the dataset in a gallery.
Change metric
to blur
or dark
to view blurry and dark images.
fd.vis.stats_gallery(metric='bright')

To get a DataFrame with statistical details for each bounding box image.
fd.img_stats()
index | img_w | img_h | unique | blur | mean | min | max | stdv | file_size | contrast | col_x | row_y | width | height | label | crop_filename | filename | is_valid | |
---|---|---|---|---|---|---|---|---|---|---|---|---|---|---|---|---|---|---|---|
0 | 0 | 326 | 259 | 256 | 392.7757 | 169.9534 | 0.0 | 255.0 | 50.3176 | 23168 | 1.0 | 0 | 73 | 272.078 | 185.083 | Turmeric Powder | work_dir/crops/DASH-DIET-101-4trainorganic-dry-turmeric-haldi-powder-also-known-as-curcuma-longa-linn-selective-focus-spice-pile-bowl-whole-191159061_jpg.rf.1adae99b28bbcbc9b808181f088bbbcf.jpg_0_73_272_185.jpg | DASH-DIET-101-4/train/organic-dry-turmeric-haldi-powder-also-known-as-curcuma-longa-linn-selective-focus-spice-pile-bowl-whole-191159061_jpg.rf.1adae99b28bbcbc9b808181f088bbbcf.jpg | True |
1 | 1 | 416 | 320 | 256 | 1337.6874 | 183.2935 | 0.0 | 255.0 | 66.9546 | 37378 | 1.0 | 42 | 0 | 358.150 | 267.799 | Turmeric Powder | work_dir/crops/DASH-DIET-101-4trainpci0920-Chemisphere-609811234-900_jpg.rf.1b8cb16482196777ebac596f522a2147.jpg_42_0_358_267.jpg | DASH-DIET-101-4/train/pci0920-Chemisphere-609811234-900_jpg.rf.1b8cb16482196777ebac596f522a2147.jpg | True |
2 | 2 | 187 | 268 | 256 | 623.9651 | 160.2206 | 0.0 | 255.0 | 79.8649 | 17050 | 1.0 | 10 | 192 | 148.320 | 223.180 | Turmeric Powder | work_dir/crops/DASH-DIET-101-4trainpci0920-Chemisphere-609811234-900_jpg.rf.1b8cb16482196777ebac596f522a2147.jpg_10_192_148_223.jpg | DASH-DIET-101-4/train/pci0920-Chemisphere-609811234-900_jpg.rf.1b8cb16482196777ebac596f522a2147.jpg | True |
3 | 3 | 360 | 319 | 256 | 730.4841 | 156.3619 | 0.0 | 255.0 | 55.2961 | 42525 | 1.0 | 0 | 135 | 300.233 | 229.676 | Turmeric Powder | work_dir/crops/DASH-DIET-101-4trainOIP-3-_jpg.rf.1c1b462382e0b3876ee2cbe6b5cc461c.jpg_0_135_300_229.jpg | DASH-DIET-101-4/train/OIP-3-_jpg.rf.1c1b462382e0b3876ee2cbe6b5cc461c.jpg | True |
4 | 4 | 266 | 48 | 256 | 178.8866 | 172.8911 | 0.0 | 255.0 | 57.3904 | 4155 | 1.0 | 0 | 0 | 222.000 | 40.292 | Turmeric Powder | work_dir/crops/DASH-DIET-101-4trainOIP-3-_jpg.rf.1c1b462382e0b3876ee2cbe6b5cc461c.jpg_0_0_222_40.jpg | DASH-DIET-101-4/train/OIP-3-_jpg.rf.1c1b462382e0b3876ee2cbe6b5cc461c.jpg | True |
Mislabels
fd.vis.similarity_gallery(slice='diff')

Wrap Up
That's it! We've just conveniently surfaced many issues with this dataset by running fastdup. By taking care of dataset quality issues, we hope this will help you train better models.
Questions about this tutorial? Reach out to us on our Slack channel!
VL Profiler - A faster and easier way to diagnose and visualize dataset issues
The team behind fastdup also recently launched VL Profiler, a no-code cloud-based platform that lets you leverage fastdup in the browser.
VL Profiler lets you find:
- Duplicates/near-duplicates.
- Outliers.
- Mislabels.
- Non-useful images.
Here's a preview of VL Profiler.
Free Usage
Use VL Profiler for free to analyze issues on your dataset with up to 1,000,000 images.
Not convinced yet?
Interact with a collection of dataset like ImageNet-21K, COCO, and DeepFashion here.
No sign-ups needed.

Updated over 1 year ago